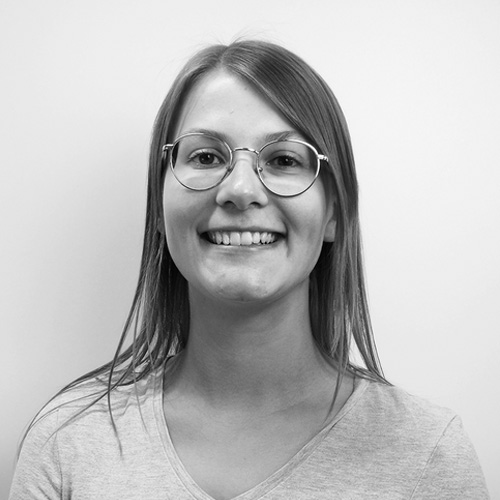
Pauline Nissen
Ethical AI Lead
As AI systems become increasingly prevalent in organisations and society, their limitations and risks are receiving more attention. Many newspaper headlines emphasise the dangers of biassed AI decisions that can potentially harm individuals. For example, in image generation tools like MidJourney, the prompt “an Indian person” often results in images of an old man with a beard, which misrepresents the diversity of the Indian population. In another context, predictive policing tools have been shown to exhibit significant racial bias, leading to disproportionately higher surveillance and policing of minority communities. This can perpetuate systemic discrimination and result in unfair treatment based on race.
Commonly, fairness is associated with avoiding discrimination based on protected characteristics such as gender or race. However, the definition of fairness differs across disciplines: law sees fairness as preventing discrimination through regulation, social science focuses on social dynamics and power imbalances, quantitative fields treat fairness as a mathematical problem, and philosophy links fairness to moral rightness and justice. This diversity in definitions contributes to confusion in applying fairness to AI systems.Moreover, fairness is inherently subjective, shaped by cultural, social and individual experiences. For example, a qualified woman facing gender discrimination in her tech career, supports her company's gender equity policy as necessary for fairness and equal opportunity in leadership roles. However, some male colleagues, who have not experienced such discrimination, view the policy as unfair preferential treatment. Although we could have dedicated an entire article solely to exploring the definition of fairness, it remains important when developing AI systems to choose one definition of fairness that should be commonly used and ensure it is implemented throughout all stages of AI development, as recommended by the High-Level Expert Group on AI. However, choosing a definition of fairness is not straightforward, as what is considered fair in one context may be perceived as unfair in another. Therefore, selecting an appropriate fairness definition for an AI project requires a collaborative and multidisciplinary approach. Input from experts, such as ethicists, legal advisors, and AI engineers, is necessary to ensure the definition is comprehensive and widely supported. It is also important to remember that regardless of the diversity of definitions available, the chosen fairness definition must comply with equality, non-discrimination and human rights laws.
A definition of fairness should also go beyond merely describing the concept; it should also involve measuring fairness. The first step in enhancing fairness is to assess the fairness of an AI system and then make adjustments to improve it. Different methods can be used to measure fairness, each with its own strengths and weaknesses. The choice of the method will depend on the context and often involve trade-offs.Fairness metrics are typically divided into group fairness and individual fairness. Group fairness ensures fair treatment across different groups by comparing model performance across them, whereas individual fairness ensures that individuals with similar qualifications receive similar outcomes. The list below is not exhaustive but consists of just examples of fairness metrics.
Demographic parity states that the selection rate should be the same across different groups of people. Simply put, sensitive attributes, such as gender, should not be the driving factor of the AI system. For example, in an AI hiring system evaluating 100 candidates, with 60 men and 40 women, demographic parity would be achieved if the hiring rate is 30% for both men (i.e. hiring 18 men) and women (i.e. hiring 12 women). However, demographic parity does not consider other relevant factors, such as qualifications, which may lead to unfair outcomes.
Equalised odds states that AI predictions should be equally accurate for different groups. This means the true positive rate and false positive rate should be the same for each group. In other words, the chances of making a correct or incorrect positive prediction should be the same for both protected and privileged groups.
Individual fairness means treating similar individuals in a similar way. For example, in a hiring AI system, two candidates with similar qualifications and experience should have the same chance of being hired.
Counterfactual fairness means that AI prediction should remain the same if a sensitive attribute were different. For example, in a hiring AI system, if a male candidate is selected for a job position, a female candidate with the same qualifications, experience, and skills should also be selected.
Unsure which fairness metric to choose for your AI project? Check out this decision tree, which guides you through a series of questions to identify the most suitable fairness metric for your AI project.
We cannot discuss fairness in AI systems without addressing the issue of bias. But what is bias, exactly? Its definition has roots in statistics, where it refers to a systematic error that results in incorrect or skewed results. In everyday terms, bias is often associated with discrimination, which implies unfair treatment based on certain attributes such as age or gender. For example, an AI system trained primarily on resumes from tech hubs like Silicon Valley may exhibit bias by favouring experiences typical of that region, which can lead to discrimination against qualified candidates from other areas.The major risk with AI systems is that they can perpetuate or even amplify existing biases. This happens because AI systems learn from the data they are trained on. If this data contains biases, the AI is likely to replicate and potentially intensify these biases in its decisions. This issue is known as algorithmic bias. However, other types of bias can also arise during the lifecycle of an AI system, including:
Historical bias: This happens when data used to train AI reflects old prejudices or unfair practices. For example, if past hiring records show a preference for certain groups of people, an AI trained on this data might continue to favour those groups, repeating past injustices.
Sample bias: This occurs when the data used to train an AI isn’t representative of the whole population. For instance, if an AI is trained mostly on data from city dwellers, it might not work well for people living in rural areas.
Selection bias: This happens when some data points are chosen in a way that skews the results. For example, if an AI system is trained only with data from a specific region, it might not make accurate predictions for people from other regions.
Bias is widespread in society, not just within AI systems. For example, in hiring practices, bias can emerge when recruiters prefer candidates from certain universities over others, despite having equal qualifications. Fundamentally, bias is built into our world and society. While we can't easily eliminate societal bias, we can take steps to remove bias from our data, models, and human review processes.
De-biasing methods are often proposed to address issues of bias. These methods assume that undesirable bias or inequality can be separated from legitimate or acceptable biases. They aim to remove this undesirable bias from both the data and the model. However, in practice, distinguishing between legitimate drivers (e.g., recidivism risk) and those influenced by demographics (e.g., gender, race) is difficult. Incorrect application of de-biasing can also reduce model accuracy and can even harm the people they intend to protect.
While de-biasing methods may not be perfect, addressing bias in AI requires a comprehensive approach that can be summarised in four points:
1. Awareness: Raising awareness among developers and stakeholders about bias is important. Understanding its sources and impacts is crucial for effective mitigation.
2. Feature selection: Identifying and carefully managing protected features such as age, race, sex, and ethnicity, as well as proxies for these characteristics. It also involves considering how non-protected features, like income or credit score, might indirectly reflect protected attributes.
3. Fairness as an optimization criterion: Ensuring that fairness is considered alongside model performance during development helps create models that are both accurate and equitable.
4. Broader AI governance: Addressing bias should be part of a wider AI governance strategy that includes ethical guidelines, regulatory compliance, and accountability mechanisms.
Addressing fairness in AI is complex and requires careful consideration. As AI becomes more widespread, there is growing concern about its potential to reinforce stereotypes and produce unfair results. Defining fairness can be challenging due to the diversity of fairness. So it's important to involve a range of experts in choosing a definition while ensuring compliance with current laws. Improving fairness involves using metrics to measure and enhance it over time. To manage bias in AI, we should focus on raising awareness, carefully managing both protected and non-protected features and proxies, making fairness an optimization criterion, and adopting strong governance practices. At ML6, we strive to create fair AI solutions by detecting and reducing bias and ensuring our data is diverse and representative to prevent discrimination.