June 19, 2023
PEFT (Parameter-Efficient Fine-Tuning)

Navigating the Parameter-Efficient Fine-tuning (PEFT) landscape can seem like a daunting task. As AI and machine learning continue to evolve, it becomes increasingly important to understand the different methods available for fine-tuning Large Language Models (LLMs) and when to use them.In this overview, we dive deep into four prominent PEFT methods: Prompt Tuning, LoRA (Low-Rank Adaptation), Adapters, and Prefix Tuning. Each slide explains what the method is and when it's best to use it.Remember, choosing a PEFT method is all about aligning with your objectives. Whether you're aiming for diverse output, task-specific attention patterns, multiple tasks on the same model, or modifying learned representations, there's a PEFT method for you. Scroll down to expand your knowledge on PEFT methods and make an informed decision next time you need to fine-tune an LLM!
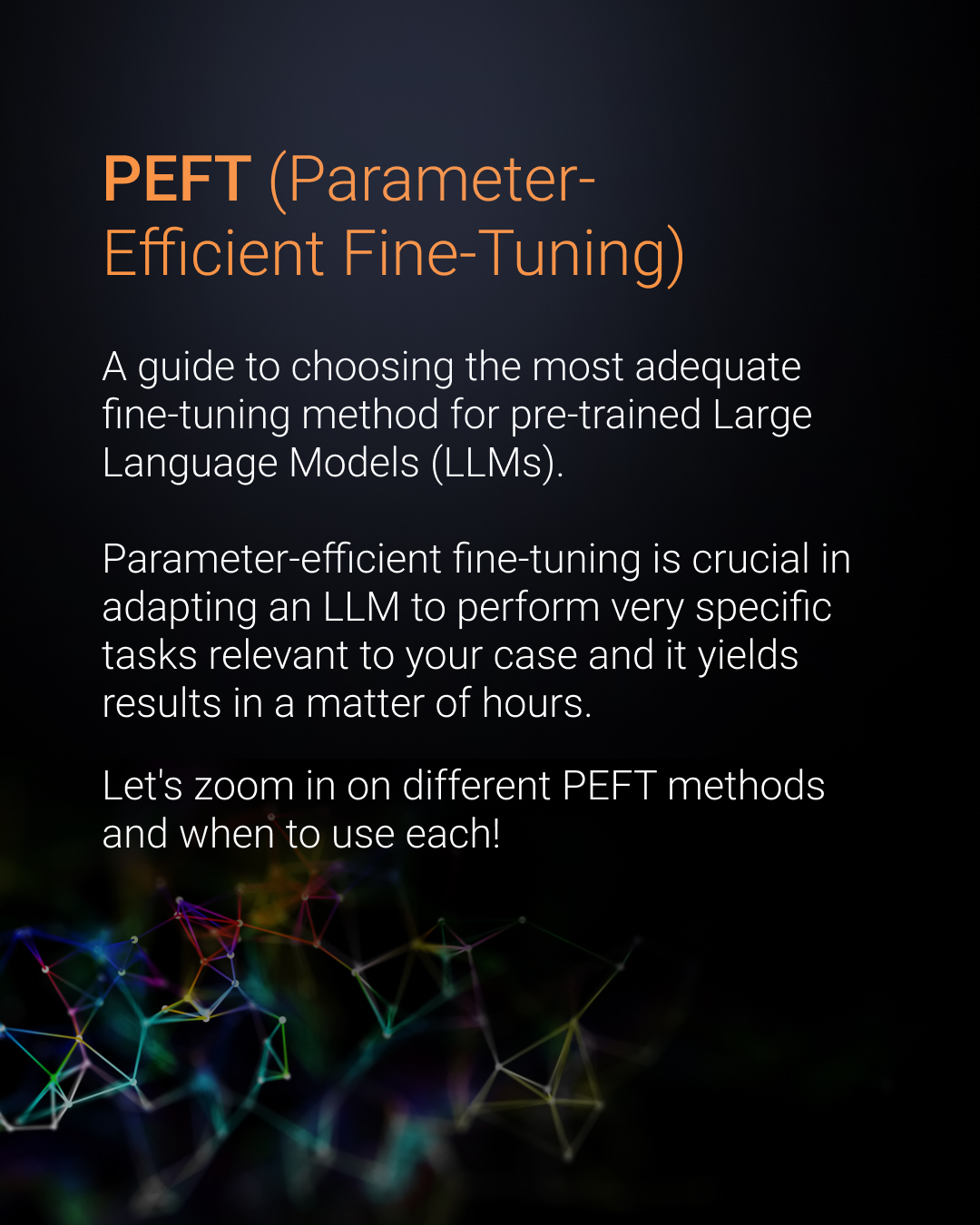
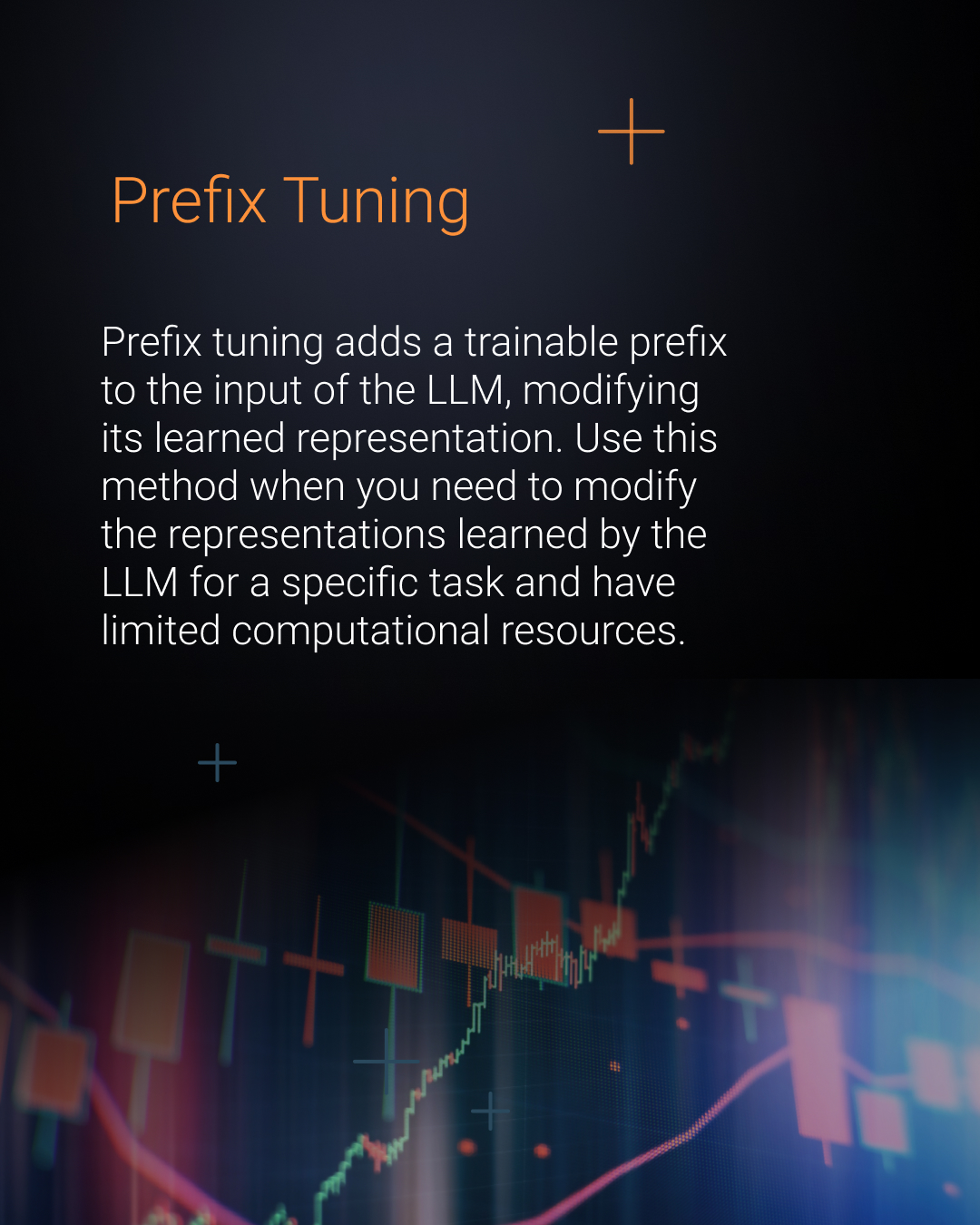

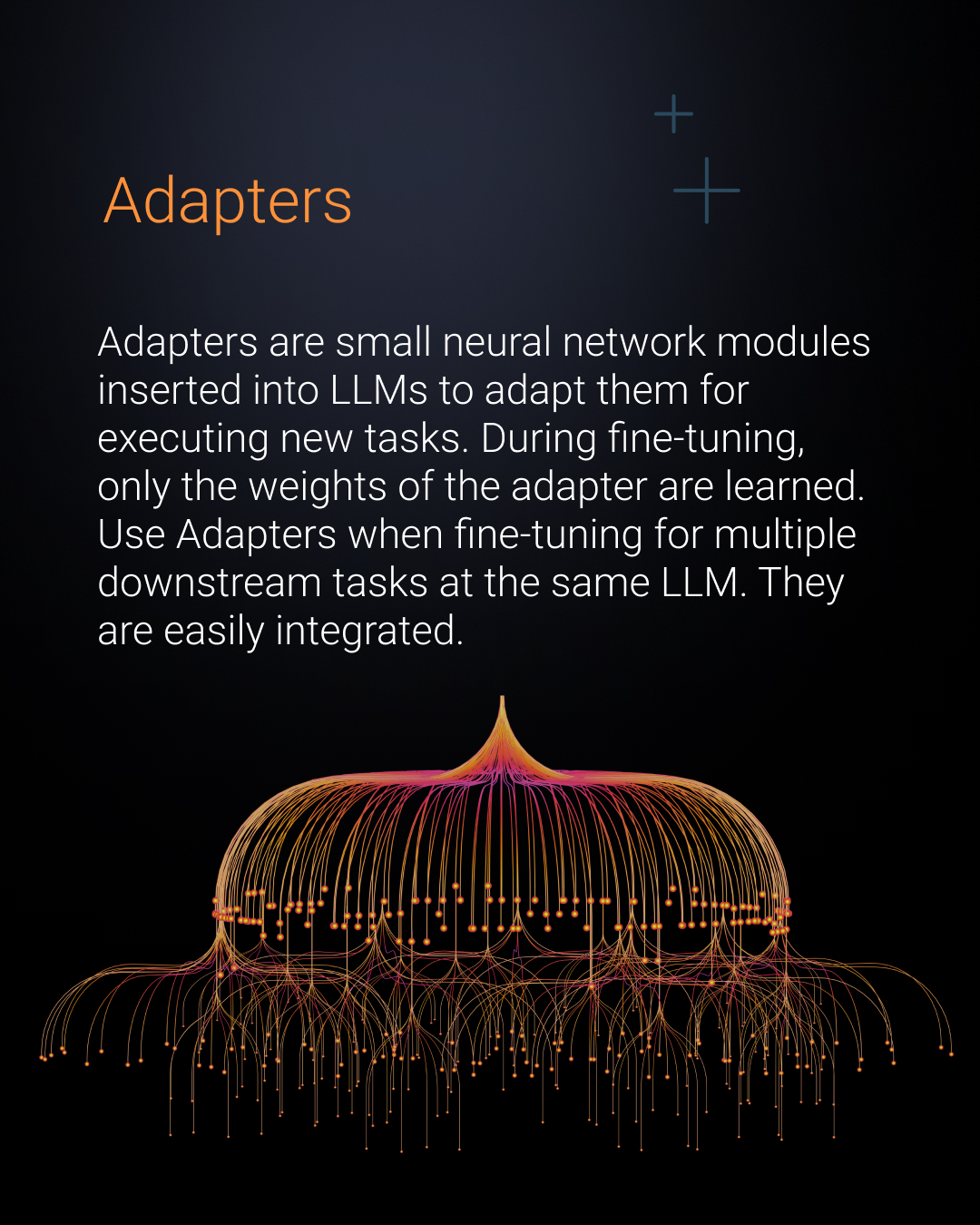

Blogposts
State of the LLM: Unlocking business potential with Large Language Models: https://bit.ly/3Wqa3QL
Low Rank Adaption: A technical deep dive: https://lnkd.in/ek478dSs
Papers
Adapters: https://lnkd.in/epXRCzRN
LoRA: https://lnkd.in/eFGq3yZW
Prefix-Tuning: https://lnkd.in/eJ9ixFpk
Prompt Tuning: https://lnkd.in/ezB5zM8Q
Repos
LoRA: https://lnkd.in/exAMvMfG