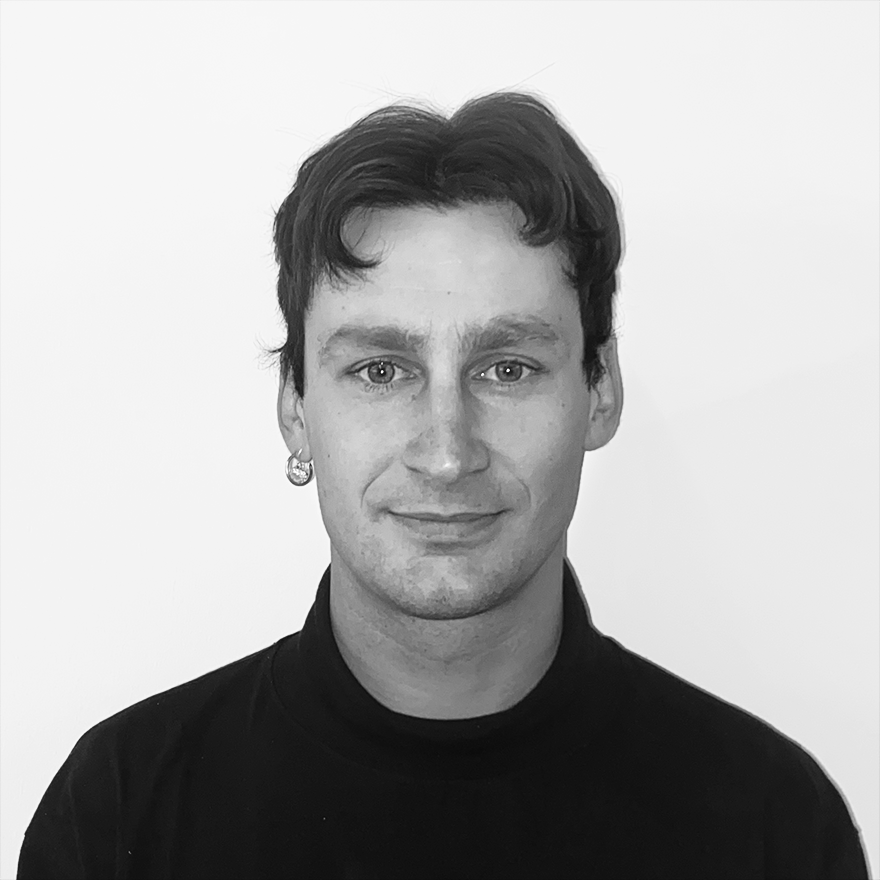
Nicholas Sujecki
Machine Learning Engineer
With recent developments, AI has transformed customer interactions, automated content creation, and facilitated services like legal and educational assistance, offering businesses unparalleled opportunities for growth and efficiency. However, these advancements come with significant risks. From mistakenly guaranteeing airfare discounts or pricing errors - like selling a car for $1 - to disseminating false, offensive, or even dangerous advice, lapses in AI judgment pose serious challenges. These errors are not only concerning for users but also present significant risks for companies, including potential legal liability or reputational damage, making it important to understand the limitations of AI solutions and how they occur.
So, how can you ensure that your next AI initiative does not end up causing a media scandal or a costly blunder? The first step is to understand the potential AI risks. Understanding why these errors occur is not always straightforward, but recognizing key areas of concern can help mitigate the potential impact on your business. Below are some of the primary risks that businesses must consider.
The list above is not exhaustive, and there are several frameworks available to help you identify AI risks, such as the Assessment List for Trustworthy Artificial Intelligence (ALTAI) or the Artificial Intelligence Risk Management Framework.
Additionally, not every risk will be relevant to your organization. Depending on the type of AI system you are using or developing, some risks may be more likely to arise or have a greater impact on your business. Therefore, when assessing the ethical risks of your AI system, it is important to evaluate both the likelihood and potential impact of each risk on your organization. While ideally, all risks would be managed, realistically, one must prioritize those with the highest impact and likelihood. Conducting such a risk assessment requires expertise in AI system design, the ethical challenges they present, and legal requirements. ML6 can support you in assessing these risks through detailed risk analysis or by offering training to share our knowledge with your team. You can learn more about our services here.
Once the risks have been identified and assessed, the next step in managing your AI system's risks is to choose an appropriate strategy and implement a corresponding mitigation plan. There are various strategies to consider, including accepting the risks, reducing them, or avoiding them altogether, which may involve modifying certain AI functionalities. The latter often arises when the AI solution's design fails to comply with relevant regulations, such as the AI Act. Mitigation plans can take different forms, ranging from organizing training to raise awareness of potential risks (e.g., addressing misinformation and hallucinations) to incorporating human oversight in critical decision-making processes (e.g., addressing failures of autonomy). Our risk management article can give you a deeper overview of frameworks you can use to mitigate AI risks.
From a regulatory perspective, we have observed that the AI Act addresses many of the ethical risks discussed here. Companies developing high-risk AI systems (i.e. AI systems that pose risks to safety, fundamental rights, and health) must comply with various requirements, including the implementation of mitigation plans for different risks. For example, one key requirement is "Data governance", which emphasizes the importance of using high-quality data in AI solutions. This means that training, testing, and validation datasets must be relevant, representative, complete, and as error-free and unbiased as possible, directly addressing the risks of bias and discrimination. Another important requirement for high-risk AI systems involves human oversight in critical business decision-making, which helps mitigate the risks associated with failures of autonomy.
While the AI Act is still evolving and more guidelines are expected, we should not wait for regulations to become clearer. We can already take steps to mitigate these risks, such as implementing guardrails.
Responsible control mechanisms, or guardrails, can be likened to the protective barriers along a winding mountain road. These barriers do not hinder progress; instead, they prevent vehicles from veering off-course, ensuring a safe journey even on the most unpredictable paths. Similarly, in the domain of AI, guardrails serve as a safety framework. They provide a layer of protection that ensures AI-generated content remains accurate, ethical, and legally compliant, enabling innovation to flourish while minimizing risks and safeguarding users.
Guardrails adhere to principles of:
Guardrails can be seen as critical context-specific safeguards that enhance and guide the functionality of AI solutions. However, they are more than just protective measures, they also reflect your organization’s commitment to ethical and responsible AI deployment. By ensuring that AI solutions are aligned with safety, fairness, and transparency standards, you not only mitigate risks but also demonstrate accountability to stakeholders, regulators, and consumers, reinforcing trust and confidence in your AI-driven initiatives.
In this section, we will focus on guardrails from a functional perspective. For a more technical explanation, you can read our article that dives into their deeper technicalities or try our interactive challenge where you can experiment with breaking the AI system yourself.
From a functional perspective, guardrails can be divided into three main categories:
These guardrails focus on managing the data entering the AI system. They can be used to curate data, ensuring the training datasets don’t contain biases, protect personal information by keeping it anonymous, or validate inputs to prevent malicious or nonsensical data from entering the AI system.
These mechanisms focus on controlling the outputs generated by the AI system. They can help moderate content to prevent offensive or harmful material from being produced, provide explanations for how the AI makes decisions, and create feedback loops that allow the system to continuously improve its outputs over time. These guardrails can range from simple functions that check language use to more complex AI models that guide and refine the system's results.
These guardrails operate at the broader system level, ensuring that AI aligns with your business, ethical, and legal requirements. This can include "human-in-the-loop" solutions, where human oversight is maintained for critical decision-making, or other monitoring systems to protect against malicious attacks and ensure the AI is performing as expected.
Managing AI risks is a complex challenge, even with the implementation of guardrails. Several factors contribute to the persistence of these difficulties.
AI models are not perfect. Due to their underlying architecture, most AI models have gaps in knowledge related to broader context and ethics, which can result in the generation of confident but inaccurate responses, often referred to as "hallucinations". These models, including large language models, are trained on vast amounts of data, the quality and accuracy of which may not always be guaranteed. Despite their impressive capabilities, many large language models have been shown to underperform in reasoning tasks without proper guidance, highlighting the limitations of current AI systems.
Society is not perfect, and this imperfection is reflected in the data used to train AI models. Since data mirrors societal structures, historical biases related to race, gender, and other stereotypes can be inadvertently emulated by models trained on such information. Additionally, users can and will deliberately misuse AI systems for personal gain, further complicating the issue. Psychological biases also play a role, making it difficult for individuals to dismiss malicious or incorrect information, even after it has been disproven. Studies show that attempts to correct disinformation can often have varying degrees of success and, in some cases, may actually reinforce existing beliefs, highlighting the challenges of combating misinformation.
Understanding these limitations, both within and outside of AI models, is important for using AI ethically and minimizing potential negative impacts. Guardrails are an effective mitigation tool, offering practical context to understand your model’s limitations. However, they are not foolproof, and attackers will always find ways to exploit vulnerabilities. Ensuring compliance with EU AI Act is an important step in mitigating AI misuse, but businesses must also look beyond these regulations to consider what further actions can and should be taken. If you want to ensure your risk management processes are future-proof against potential legal liabilities and reputational damage, while strengthening your leadership in the industry, don’t hesitate to contact us.
Good luck out there,
ML6