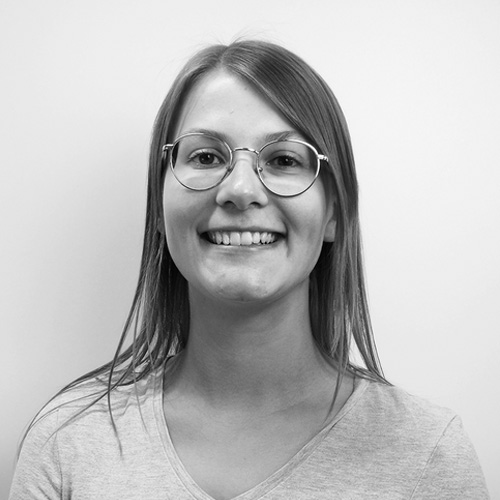
Pauline Nissen
Ethical AI Lead
Since the launch of ChatGPT, AI tools have become much more widely used in many organisations. This technology opens up many new opportunities, such as automating customer service or improving content creation. However, it also introduces significant risks. Headlines in press articles frequently mention concerns such as deep fakes, ethical dilemmas around AI replacing artists, legal disputes over copyright between LLM providers and media companies, and privacy issues.
The growing use of Generative AI tools has increased the need for strong AI Governance practices in organisations. Many organisations are now drafting policies concerning the use of ChatGPT in their business activities to prevent leaks of confidential data. Internally, they share guidelines to ensure employees can adopt the technology while minimising risks.
However, AI Governance itself is not a new concept. AI Governance refers to the set of frameworks, policies, and processes to ensure that AI systems are designed, deployed, used, and managed to maximise benefits while preventing harm. In practice, AI Governance operates on multiple levels, from formulating AI policy to raising awareness about AI among employees through internal training programs.
The initial step in implementing AI Governance is to create an overview of the organisation's current and future AI projects. This provides insights into AI usage, associated risks, and compliance with legal and ethical standards. One approach is to use risk management frameworks to identify potential risks and develop mitigation strategies. This process can help identify recurring risks and integrate global policies into practical guidelines. In this blog post, we will specifically focus on risk management frameworks and share our insights.
When searching for “risk management frameworks for AI” on Google, you will quickly discover that such frameworks are not uncommon. Many esteemed research organisations have published their own frameworks on AI Governance and Risk Management. Instead of starting from scratch, we have built our Risk Management Framework based on insights from these existing frameworks and our hands-on experience with client projects. This section will go deeper into the existing frameworks used for risk management.
When considering risk management frameworks, it's important to take their varying nature and emphasis into account. Some frameworks focus on risk identification, offering tools for risk assessment and evaluation, while others focus on mitigating risks, providing guidelines for addressing specific risks. In this blog post, we consider risk management frameworks that combine both risk identification and risk mitigation efforts.
In 2019, the High-Level Expert Group on AI (AI HLEG) released ethics guidelines for Trustworthy AI and an Assessment List for Trustworthy Artificial Intelligence (ALTAI). The Assessment List includes a series of questions to evaluate potential risks across seven dimensions: human oversight, technical robustness, privacy and data governance, transparency, diversity and fairness, societal well-being, and accountability. These questions encourage looking at AI risks from all angles, considering things you might not think of at first. But answering each one can be time-consuming, and may not be necessary for AI projects with minimal risks. Also, because the guidelines were published in 2019, they don't cover new developments like Generative AI or the EU AI Act.
More recently, in April 2024, the National Institute of Standards and Technology (NIST) introduced the “Artificial Intelligence Risk Management Framework: Generative Artificial Intelligence Profile”. The framework is an important resource for organisations seeking to identify and mitigate risks associated with Generative AI. It specifically targets risks, like hallucinations, harmful content, data privacy, intellectual property, and toxicity. The framework also provides a list of guidelines to address these risks, focusing on governance, mapping, measurement, and management processes. Thus, the focus is more on procedural aspects, like documentation, transparency and metrics, rather than on technical implementation. Since NIST is an American organisation, the framework doesn’t contain a direct mapping with the requirements of the EU AI Act. However, many recommendations are still applicable and may overlap with those of the AI Act, although a direct mapping between the two has not been undertaken.
The Alan Turing Institute released a series of 8 workbooks to help the public sector apply AI Ethics and Governance Principles in designing, developing, and deploying AI solutions safely. The framework introduces AI and ethics concepts and includes many practical templates and activities for organisations to identify risks. Researchers advocated for a Process-Based Governance approach to integrate principles, like accountability, fairness, explainability, and data stewardship throughout every stage of an ML project lifecycle.
In Flanders, Digitaal Vlaanderen also published Guidelines for the use of publicly accessible Generative AI. In addition to these guidelines, they provide examples demonstrating how this technology can be used by employees of the Flemish Government, along with tips for writing prompts.
At ML6, we have conducted multiple risk assessments and offered recommendations to our clients for addressing these risks. In this section, we share our practical insights and tips gained from these hands-on experiences.
Risk management frameworks constitute only a part of an AI Governance framework. Given the potential opportunities and risks presented by new technological advancements, it's important to adopt a proactive approach towards AI governance. AI Governance not only enhances clarity for employees but also ensures that risks are mitigated before any potential reputational damage occurs. If you're interested in exploring how AI Governance could be implemented to meet the specific needs of your organisation, feel free to reach out.